The Evolution of AI in Legal Tech: Past, Present, and Future
From pattern recognition to large language models, learn how AI is transforming legal tech and what the future holds.
The excitement surrounding AI's advancements has reached a fever pitch in the last eighteen months. Everywhere I turn, there's news about how AI can do something new and amazing. Not a day goes by, and another startup has raised the stakes before I can even digest yesterday's news. Then, only a few days later, I'm seeing some of these things showing up in products I use daily on the web.
I don't know about you, but this leaves me feeling like I'm not quite sure what's going on or what to expect next. As a software developer and eDiscovery professional, I've always been interested in machine learning, but all this caught me off guard. I didn't think AI would perform like this for at least another decade.
I had to sit down and think things over, review what I thought I knew about machine learning, and incorporate all these new developments to develop a new mental model. What lies ahead? Not just in general but especially as it relates to eDiscovery.
I thought it would be best to lay out my thoughts as a review of the progression of AI technology: its past, present, and future.
The Past: Pattern Recognition
In the past, AI consisted of a small set of tools with limited capabilities. Most machine learning and AI models revolved around complex pattern recognition and classifying different things. First, it was small chunks of text, then images and faces, and then larger sets of unstructured text. From these basic building blocks, we built some pretty powerful tools for eDiscovery — tools that helped cull massive data sets for review, organize them sensibly, and quickly find things of interest in them.
Predictive Coding
Also known in the industry as technology-assisted review (TAR), machine learning algorithms predict the relevance of documents, significantly reducing manual review time. Predictive coding can prioritize documents for review or exclude large volumes of data for review altogether.
Predictive coding relies on iterative learning and feedback to improve its accuracy. The system becomes more effective at identifying relevant documents by continuously analyzing training data from human coding decisions. It's important to note that this supervised machine-learning task takes time and expertise to implement well. Review teams usually must do this training on a case-by-case basis. Despite these challenges, the legal industry has widely adopted this technology for eDiscovey. It has proven to be a valuable tool for managing large volumes of documents defensibly and efficiently.
Clustering and Categorization
By grouping similar documents, AI aids in exploring new ideas and connections related to the case that might not be immediately obvious. Clustering highlights key themes and patterns that often reveal underlying issues, potential arguments, or critical evidence previously overlooked. An excellent example of this would be the sunburst diagram used in Reveal.
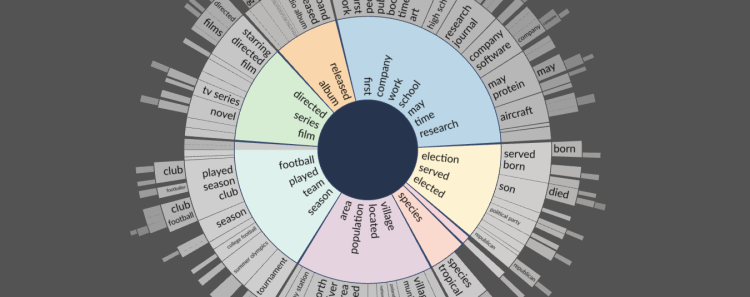
Legal teams usually need to get a 20,000-foot view of a case quickly. Since clustering is an unsupervised machine-learning task, it's possible to do without human trainers early in the process. The review team can see a broader case context and hit the ground running with that information.
Image and Object Recognition
AI-identified objects within photos are helpful for visual data analysis and finding items or people of interest in large datasets. We all saw how tagging faces on Facebook became very good at its job about ten years ago. This same technology, applied to more generalized things, now allows us to search sets of photos much more like text.
For a few years, we've used machine vision to search thousands of photos for particular objects and people. This area of machine learning is improving rapidly and will continue to see dramatic improvements.
Named Entity Recognition (NER)
NER identifies, canonicalizes, and categorizes people, times, places, and things mentioned using natural language within unstructured text. People tend to refer to all of these things in many different ways. Time references are an excellent example of how people articulate references can vary. For example, I might prefer a date format of "Month/Day/Year," and someone else might use "Month Day, Year." To further complicate this, we have the European syntax of "Day/Month/Year," and sometimes, people abbreviate the month in "Month Day, Year." That is the tip of the iceberg regarding the complexities of recognizing date references. NER enhances clustering and categorization efforts by automatically extracting, normalizing, and linking these entities. It groups similar documents based on identified entities. In addition, it can help create visualizations like structured graphs that reveal underlying issues, potential arguments, and key evidence.
The Present: LLMs and Generative AI
The emergence of large language models (LLMs) has dramatically changed things. Simple forms of classifiers and natural language processing (NLP) have been used in the past to accomplish things like sentiment analysis, semantic search, and document summaries. But often, these features seemed like novelties and didn't perform as advertised. The quality of results one can expect using LLMs has genuinely changed, and AI can now be relied upon to facilitate real workflows for these use cases.
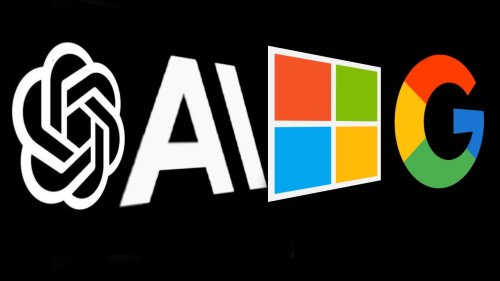
Sentiment Analysis
Sentiment analysis has been around in one form or another for a while. It is about figuring out the emotional tone in text, like whether a review is positive or negative. LLMs are great for this because they're trained on lots of text, making them good at understanding human language. They can analyze all kinds of text, from tweets to reviews, and handle large amounts of data. Sentiment analysis has improved dramatically since the introduction of LLMs.
In eDiscovery, analysts can use sentiment analysis to quickly gauge the emotional tone of emails, documents, and communications. Sentiment analysis helps identify potential evidence by detecting signs of deception or distress and prioritizing documents that may be more relevant to the case.
Semantic Search
We all remember the bad old days of crafting complex keyword lists and using Boolean operators to find information. This approach, known as lexical search, matches exact keywords without understanding the context, often leading to irrelevant results. We had to noodle many additional qualifiers to create a reasonably actionable search set.
In contrast, semantic search uses AI to grasp the meaning behind words, considering context and user intent. It delivers more accurate and relevant results by matching the overall concept of the query with content. In the discovery space, several heavy hitters like Reveal and Relativity have already implemented these capabilities into their products.
Although semantic search has existed for some time, it has taken on a new life in the era of LLMs. Companies like Elastic Search and Cohere are at the forefront of building robust semantic search models using LLMs and retrieval-augmented generation (RAG). I expect to see substantial improvements to these capabilities in most eDiscovery software suites soon.
It's worth noting that a skilled operator can perform this same function with a classic lexical search. In this case, the human operator is the semantic component of the system. AI-facilitated semantic search is particularly useful for less skilled users who want to perform their own searches. Access to full-time seasoned eDiscovery professionals who know how to use their axe is still a preferable option in general.
Generative Summaries
Generative summaries use AI to create concise and coherent summaries of large documents or datasets. Obvious use cases include summarizing legal briefs, pleadings, emails, meeting minutes, text threads, and contracts. These summaries help users cut through large review sets, saving time and money.
The Future: Everything, Everywhere, All At Once
One of the most potent ways AI will change not just eDiscovery but all your interactions with computers is one you won't even notice. Programs that are engineered properly just work, and users are not necessarily impressed. They move on with their day without a second thought.
It has already been happening all around you: AI weaving its way into everything you use. Like all good software features, these features do their job and get out of the way. Your day is a little easier as a result, and you can check out at 5 for happy hour.
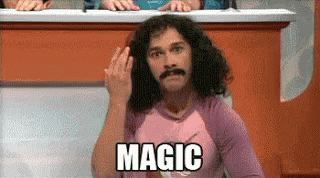
So what else can you expect?
More Refined Models
All the machine learning models mentioned will become highly refined over time. As more people use them, they will get better, thanks to the large amounts of training data these new users provide. It's a virtuous cycle, and we're only at the start of this rapid improvement phase. Each model will advance at its own pace, depending on its niche. Progress in one area won't necessarily translate directly to another.
Machine Vision
Machine Vision is an excellent example of a niche that has recently made significant strides. The latest advancements enable it to perceive and interpret more complex elements. Modern systems can detect activities, analyze sentiments, and understand spatial relationships more accurately. These enhancements allow AI to recognize human actions, such as walking or gesturing, and interpret emotional cues from facial expressions or body language. Improved spatial awareness enables AI to understand objects' relative positions and movements in a scene, providing a more comprehensive visual data analysis.
Being able to pinpoint relevant events or behaviors in surveillance footage is just one example of how machine vision will accelerate future eDiscovery work.
New User Interfaces
User experience is one of the more significant areas of speculation. Are we going to see radical new user experiences? Is the way we interact with eDiscovery software going to change fundamentally? I'm inclined to say yes. Of course, all the old modalities will still exist, but some new ones will likely emerge. To understand why I think this, it's useful to look at two examples of how new agent-based interaction can work in complex user interactions.
The first is an agent-based shopping experience recently demoed by Google using the Gemini and Vertex AI platforms. I've cued up the relevant section.
Providing visual material from a YouTube video, then asking the agent a related question or two, and getting highly relevant search results is pretty wild. The possibilities of this kind of interaction in the context of eDiscovery are compelling.
Machine vision is already moving from identifying general types of objects to specific objects like the make and model of vehicles or particular brands and models of handbags. Combining this with open-source intelligence (OSINT) to cross-reference online images and sources will save significant time and money. For instance, recognizing a Gucci bag in a video and instantly verifying it with online photos and product detail pages. The fact that it was a specific Gucci bag might have high relevance to a case and have been otherwise overlooked or difficult to identify without outside experts in fashion or retail.
The second video is a demo of Palantir AIP for defense. It immediately earned the nickname WarGPT on social media. Yes, it's exactly what it sounds like. It's an agent-based battlefield awareness and tactical command application using LLMs and real-time data to drive a chat-driven user experience.
That demo blew my mind. Imagine being able to do something similar with a corpus of discovery documents as your data source. Talking to an agent and discussing what is in the data set, finding things, reasoning about them, and developing strategies in real-time is, again, pretty wild.
AI Is Narrow, Not General
Upon review, many excellent AI features have already been slowly making their way into eDiscovery work for a long time. Maybe I shouldn't have been so surprised by the developments of the last two years. In any case, I have one final parting observation.
The features I outlined here are assistive in nature and narrow in capabilities. Each of the models I've outlined above, except for the speculation about chat-based interactions, is fairly niche. Every model has a specific task to fulfill.
People tend to conflate the idea of AI with things they may have seen in science fiction movies. Artificial General Intelligence does not currently exist in any meaningful way. It will be a while before AI can do general-purpose work of any quality, so it is unwise to try using it that way for now. Too many kids are having GPTs do their homework for them. It's an entirely abusive and stupid way to use these technologies. They usually get caught and are worse off for it, even if they don't.
I'll leave you with the cautionary tale. A high-profile lawyer for Pras Micheals, a member of the Fugees, recently tried to do the adult equivalent of having ChatGPT do his homework. He had an LLM craft his closing arguments for a high-profile case. It didn't turn out well, but it's pretty entertaining reading.
Machine learning and AI certainly portend an interesting and entertaining near future. When used wisely, these tools will provide significant power and advantages to those who adopt them early. I encourage you to embrace these technologies with a clear understanding of their capabilities... and limitations.
Cover Photo by Google DeepMind from Pexels: